SHAP on MNIST
This is an example of SHAP on image data. If using this explainer, please cite the original work: https://github.com/slundberg/shap. ShapImage
is not a model-agnostic method as ShapTabular
. It only support Tensorflow or PyTorch models.
[1]:
# This default renderer is used for sphinx docs only. Please delete this cell in IPython.
import plotly.io as pio
pio.renderers.default = "png"
[2]:
import numpy as np
import tensorflow as tf
import matplotlib.pyplot as plt
from omnixai.data.image import Image
from omnixai.explainers.vision import ShapImage
The following code loads the training and test datasets. We recommend using Image
to represent a batch of images. Image
can be constructed from a numpy array or a Pillow image. In this example, Image
is constructed from a numpy array containing a batch of digit images.
[3]:
# Load the MNIST dataset
img_rows, img_cols = 28, 28
(x_train, y_train), (x_test, y_test) = tf.keras.datasets.mnist.load_data()
if tf.keras.backend.image_data_format() == 'channels_first':
x_train = x_train.reshape(x_train.shape[0], 1, img_rows, img_cols)
x_test = x_test.reshape(x_test.shape[0], 1, img_rows, img_cols)
input_shape = (1, img_rows, img_cols)
else:
x_train = x_train.reshape(x_train.shape[0], img_rows, img_cols, 1)
x_test = x_test.reshape(x_test.shape[0], img_rows, img_cols, 1)
input_shape = (img_rows, img_cols, 1)
# Use `Image` objects to represent the training and test datasets
train_imgs, train_labels = Image(x_train.astype('float32'), batched=True), y_train
test_imgs, test_labels = Image(x_test.astype('float32'), batched=True), y_test
The preprocessing function takes an Image
instance as its input and outputs the processed features that the ML model consumes. In this example, the pixel values are normalized to [0, 1].
[4]:
preprocess_func = lambda x: np.expand_dims(x.to_numpy() / 255, axis=-1)
We train a simple convolutional neural network for this task. The network has two convolutional layers and one dense hidden layer.
[5]:
batch_size = 128
num_classes = 10
epochs = 10
x_train = preprocess_func(train_imgs)
x_test = preprocess_func(test_imgs)
y_train = tf.keras.utils.to_categorical(y_train, num_classes)
y_test = tf.keras.utils.to_categorical(y_test, num_classes)
model = tf.keras.models.Sequential()
model.add(tf.keras.layers.Conv2D(
32, kernel_size=(3, 3), activation='relu', input_shape=input_shape))
model.add(tf.keras.layers.Conv2D(64, (3, 3), activation='relu'))
model.add(tf.keras.layers.MaxPooling2D(pool_size=(2, 2)))
model.add(tf.keras.layers.Dropout(0.1))
model.add(tf.keras.layers.Flatten())
model.add(tf.keras.layers.Dense(128, activation='relu'))
model.add(tf.keras.layers.Dropout(0.1))
model.add(tf.keras.layers.Dense(num_classes))
model.compile(
loss=tf.keras.losses.CategoricalCrossentropy(from_logits=True),
optimizer=tf.keras.optimizers.Adam(),
metrics=['accuracy']
)
model.fit(
x_train, y_train,
batch_size=batch_size,
epochs=epochs,
verbose=1,
validation_data=(x_test, y_test)
)
score = model.evaluate(x_test, y_test, verbose=0)
print('Test loss:', score[0])
print('Test accuracy:', score[1])
Epoch 1/10
469/469 [==============================] - 2s 5ms/step - loss: 0.1724 - accuracy: 0.9487 - val_loss: 0.0458 - val_accuracy: 0.9855
Epoch 2/10
469/469 [==============================] - 2s 5ms/step - loss: 0.0466 - accuracy: 0.9852 - val_loss: 0.0333 - val_accuracy: 0.9885
Epoch 3/10
469/469 [==============================] - 2s 5ms/step - loss: 0.0298 - accuracy: 0.9906 - val_loss: 0.0323 - val_accuracy: 0.9891
Epoch 4/10
469/469 [==============================] - 2s 5ms/step - loss: 0.0207 - accuracy: 0.9933 - val_loss: 0.0286 - val_accuracy: 0.9903
Epoch 5/10
469/469 [==============================] - 2s 5ms/step - loss: 0.0158 - accuracy: 0.9949 - val_loss: 0.0295 - val_accuracy: 0.9907
Epoch 6/10
469/469 [==============================] - 2s 5ms/step - loss: 0.0125 - accuracy: 0.9962 - val_loss: 0.0290 - val_accuracy: 0.9904
Epoch 7/10
469/469 [==============================] - 2s 5ms/step - loss: 0.0109 - accuracy: 0.9963 - val_loss: 0.0283 - val_accuracy: 0.9902
Epoch 8/10
469/469 [==============================] - 2s 5ms/step - loss: 0.0090 - accuracy: 0.9970 - val_loss: 0.0323 - val_accuracy: 0.9903
Epoch 9/10
469/469 [==============================] - 2s 5ms/step - loss: 0.0065 - accuracy: 0.9978 - val_loss: 0.0317 - val_accuracy: 0.9912
Epoch 10/10
469/469 [==============================] - 2s 5ms/step - loss: 0.0072 - accuracy: 0.9974 - val_loss: 0.0359 - val_accuracy: 0.9915
Test loss: 0.03591015189886093
Test accuracy: 0.9915000200271606
To initialize ShapImage
, we need to set the following parameters:
model
: The ML model to explain, e.g.,torch.nn.Module
ortf.keras.Model
.preprocess_function
: The preprocessing function that converts the raw data into the inputs ofmodel
.
[6]:
explainer = ShapImage(
model=model,
preprocess_function=preprocess_func
)
We can simply call explainer.explain
to generate explanations for this classification task. ipython_plot
plots the generated explanations in IPython. Parameter index
indicates which instance to plot, e.g., index = 0
means plotting the first instance in test_imgs[0:5]
.
[7]:
explanations = explainer.explain(test_imgs[0:5])
explanations.ipython_plot(index=4)
WARNING:tensorflow:From /home/ywz/anaconda3/lib/python3.7/site-packages/shap/explainers/_deep/deep_tf.py:239: set_learning_phase (from tensorflow.python.keras.backend) is deprecated and will be removed after 2020-10-11.
Instructions for updating:
Simply pass a True/False value to the `training` argument of the `__call__` method of your layer or model.
Using TensorFlow backend.
keras is no longer supported, please use tf.keras instead.
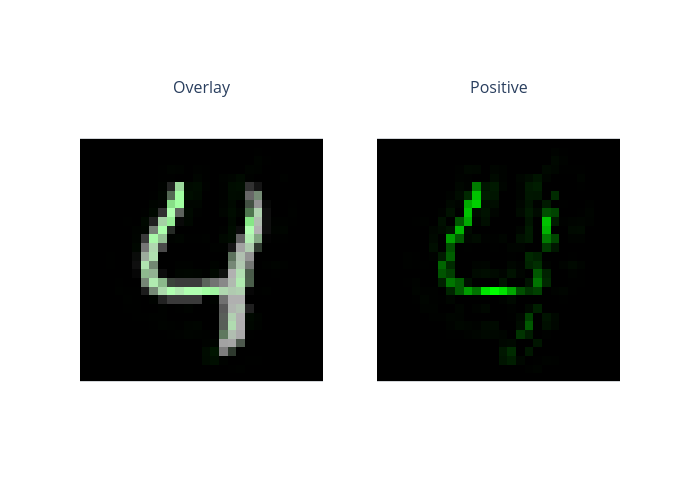