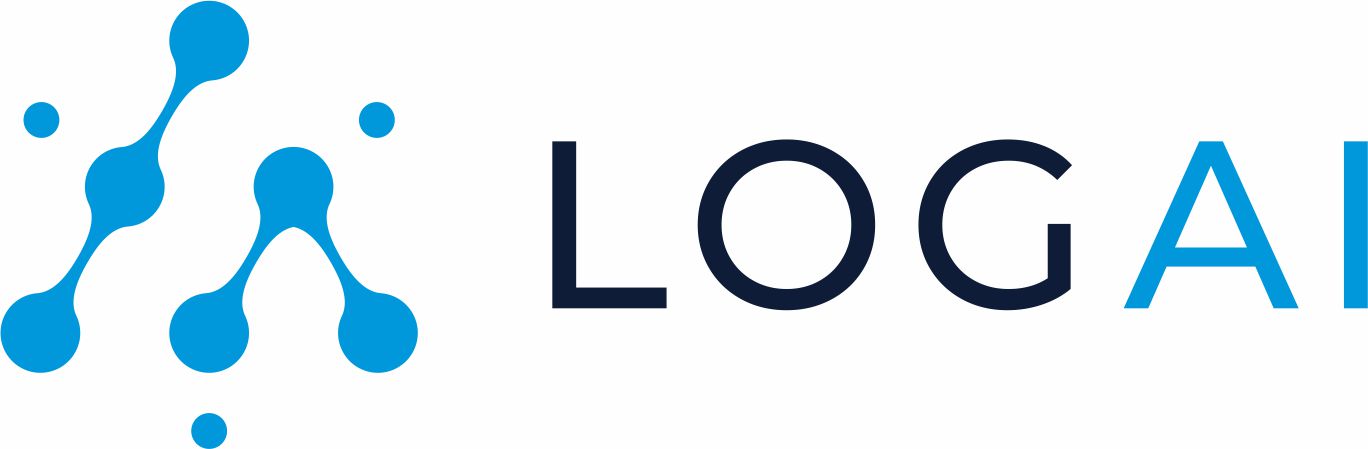
Getting Started
Installation
You can install LogAI core library using pip install
:
# Check out LogAI code repo from Github
git clone https://git.soma.salesforce.com/SalesforceResearch/logai.git
cd logai
# [Optional] Create virtual environment
python3 -m venv venv
source venv/bin/activate
# Install LogAI
pip install logai
Install Optional Dependencies
LogAI core library is light-weight with limited dependent packages installed. Users can install optional dependencies to enable extended functionalities of LogAI.
Deep Learning Log Analysis. To conduct deep learning model related tasks and run benchmarking,
please install extra requirements by pip install "logai[deep-learning]"
.
Enable LogAI GUI portal*. To use LogAI GUI portal,
please install extra requirements by pip install "logai[gui]"
.
LogAI Development. To contribute to LogAI development, build and test code changes,
please install extra requirements by pip install "logai[dev]"
.
Complete installation. you can install the full list of dependencies by pip install "logai[all]"
.
Use LogAI
Below we briefly introduce several ways to explore and use LogAI, including exploring LogAI GUI portal, benchmarking deep-learning based log anomaly detection using LogAI, and building your own log analysis application with LogAI.
Explore LogAI GUI Portal
You can also start a local LogAI service and use the GUI portal to explore LogAI.
# Check out LogAI code repo from Github
git clone https://git.soma.salesforce.com/SalesforceResearch/logai.git
cd logai
# [Optional] Create virtual environment
python3 -m venv venv # create virtual environment
source venv/bin/activate # activate virtual env
# install LogAI and GUI dependencies
pip install ".[dev]"
pip install ".[gui]"
# Start LogAI service
export PYTHONPATH='.' # make sure to add current root to PYTHONPATH
python3 gui/application.py # Run local plotly dash server.
Then open the LogAI portal via http://localhost:8050/
or http://127.0.0.1:8050/
in your browser:
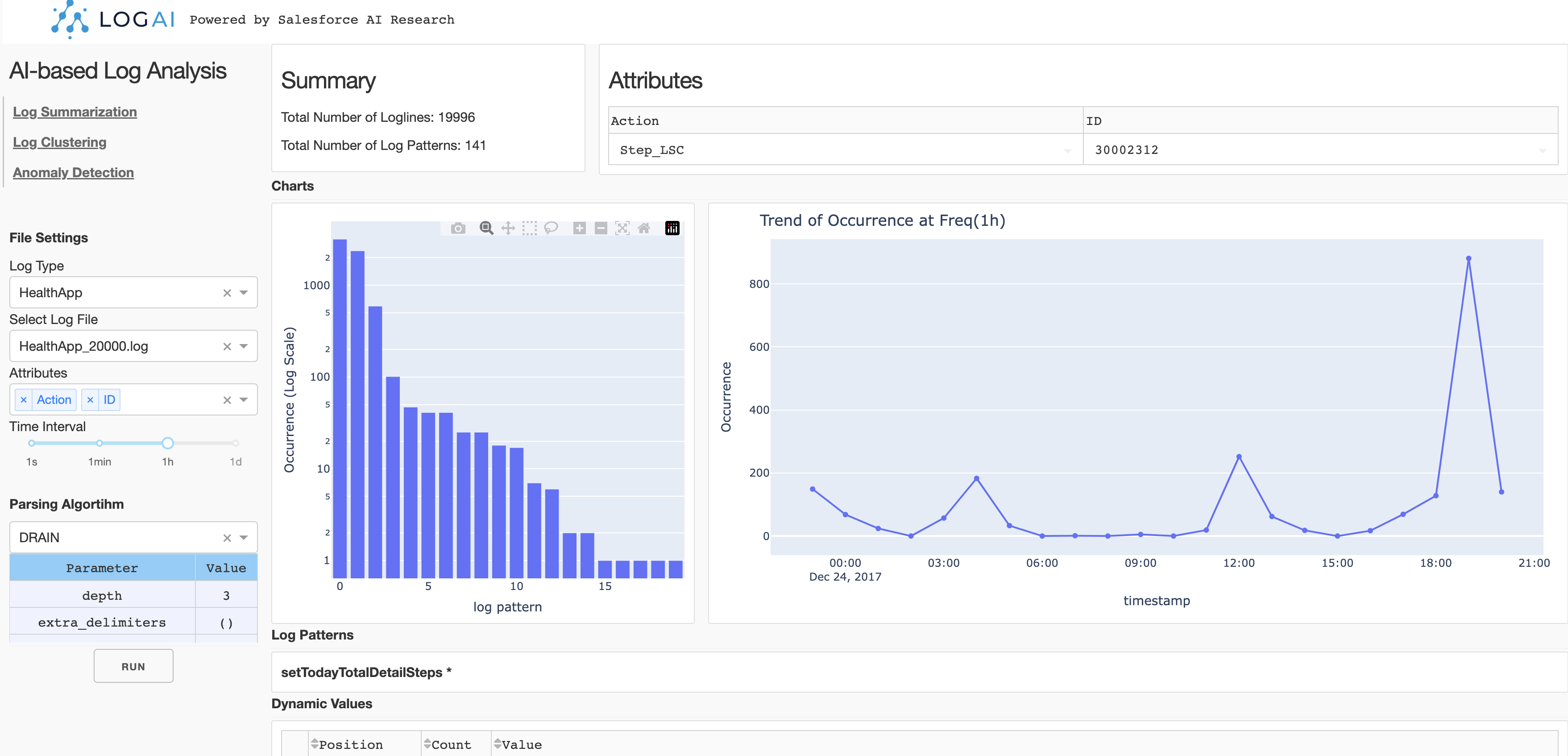
Run Simple Time-series Anomaly Detection Application
You can also use LogAI in more programtic ways. LogAI supports configuration files in .json or .yaml. Below is a sample log_anomaly_detection_config.json configuration for anomaly detection application. Make sure to set filepath to the target log dataset file path.
{
"open_set_data_loader_config": {
"dataset_name": "HDFS",
"filepath": ""
},
"preprocessor_config": {
"custom_delimiters_regex":[]
},
"log_parser_config": {
"parsing_algorithm": "drain",
"parsing_algo_params": {
"sim_th": 0.5,
"depth": 5
}
},
"feature_extractor_config": {
"group_by_category": ["Level"],
"group_by_time": "1s"
},
"log_vectorizer_config": {
"algo_name": "word2vec"
},
"categorical_encoder_config": {
"name": "label_encoder"
},
"anomaly_detection_config": {
"algo_name": "one_class_svm"
}
}
Then to run log anomaly detection. You can simply create below python script:
import json
from logai.applications.application_interfaces import WorkFlowConfig
from logai.applications.log_anomaly_detection import LogAnomalyDetection
# path to json configuration file
json_config = "./log_anomaly_detection_config.json"
# Create log anomaly detection application workflow configuration
config = json.loads(json_config)
workflow_config = WorkFlowConfig.from_dict(config)
# Create LogAnomalyDetection Application for given workflow_config
app = LogAnomalyDetection(workflow_config)
# Execute App
app.execute()
Then you can check anomaly detection results by calling app.anomaly_results
.
For full context of this example please check Tutorial: Use Log Anomaly Detection Application.
Build Customized LogAI Applications
You can build your own customized log analysis applications using LogAI. Here we show two examples:
Deep-learning Anomaly Detection Benchmarking
LogAI can be used to benchmark deep-learning anomaly detection results. A tutorial is provided for Anomaly Detection Benchmarking using LSTM anomaly detector for HDFS Dataset. More examples of deep-learning anomaly detection benchmarking on different datasets and algorithms can be found in Deep Anomaly Detection Benchmarking Examples.